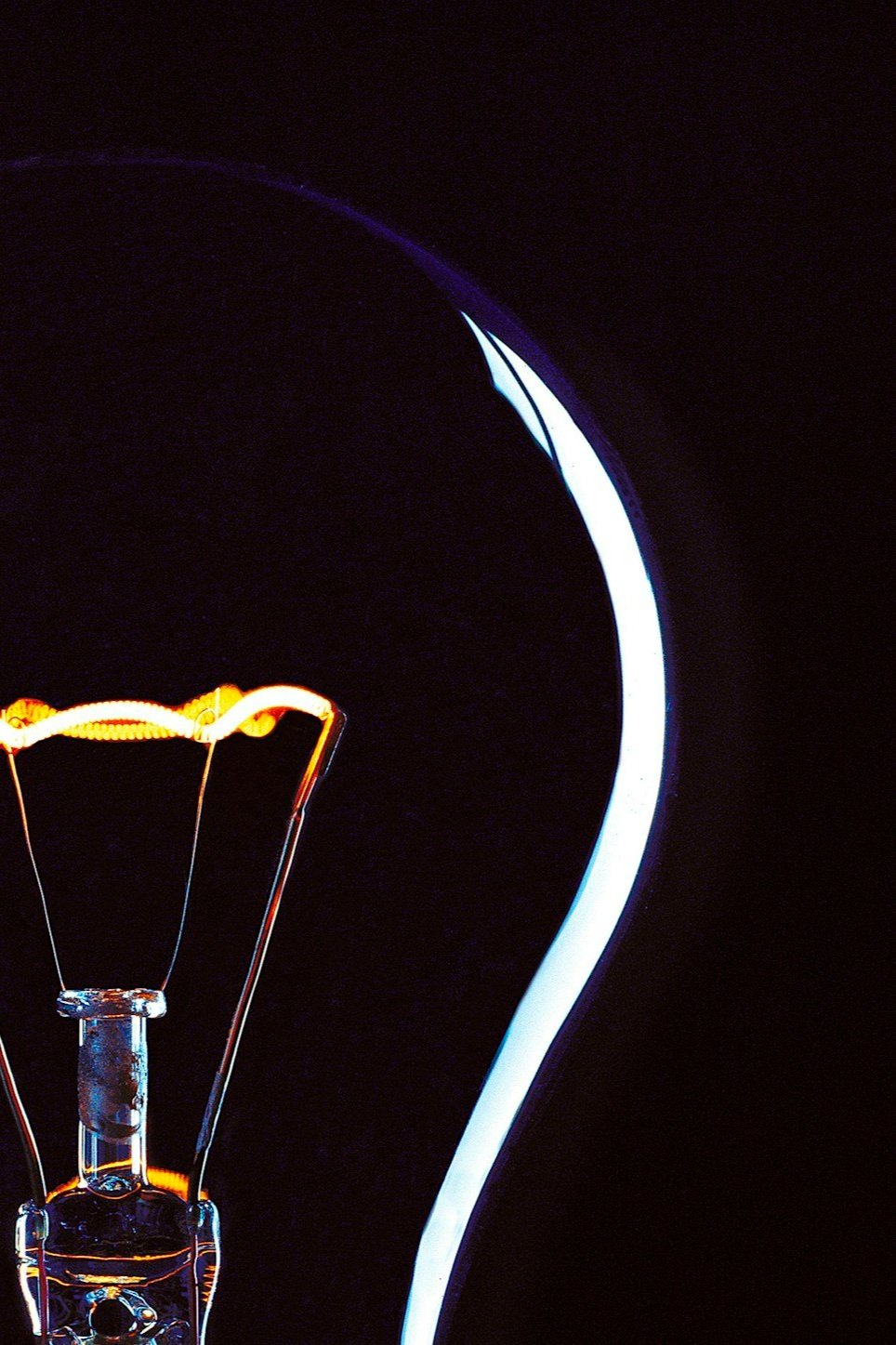
Undergraduate Research
Research with Dr. Umair Rehman
Benchmarking Text-to-Speech Models (Expected Publish Date: July 2025)
ABSTRACT
This study introduces a robust, quantitative framework for benchmarking Text-to-Speech (TTS) models, systematically evaluating their performance across three critical dimensions: intelligibility, expressiveness, and computational efficiency. Conventional TTS assessments predominantly depend on subjective Mean Opinion Score (MOS) studies, which, despite capturing human perceptual insights, suffer from inconsistent reproducibility and scalability due to listener variability. To overcome these limitations, we evaluate nine state-of-the-art TTS models, including Tacotron2 variants, FastPitch, and VITS, using a comprehensive suite of objective metrics: Word Error Rate (WER), and Character Error Rate (CER) for intelligibility, Signal-to-Noise Ratio (SNR) and pitch variance for expressiveness, and processing latency for efficiency. Leveraging a standardized dataset of 58 carefully curated prompts from the LibriVox corpus, we generate 522 diverse speech samples, which are analyzed through automated transcription with Whisper ASR and advanced spectral processing techniques. Our results expose significant trade-offs among accuracy, naturalness, and computational cost, highlighting that model performance is highly context-dependent rather than universally optimal. For instance, high-efficiency models like FastPitch excel in speed but compromise on expressive nuance, while models like Neural-HMM prioritize naturalness at the expense of processing time. This framework provides a holistic, data-driven comparison of modern TTS systems. It establishes a scalable and reproducible methodology, paving the way for standardized, future advancements in TTS evaluation and development.
Research with Dr. Tiffany Bailey and Dr. Kyle Maclean
Critical Pathways for Business Education
Presented at INFORMS Seattle 2025 Conference